Can ISO 20022 Improve Your Correspondent Banking Transaction Monitoring Outcomes?
Learn how implementing MX data can enhance AI capabilities and improve risk detection, despite the associated costs and challenges.
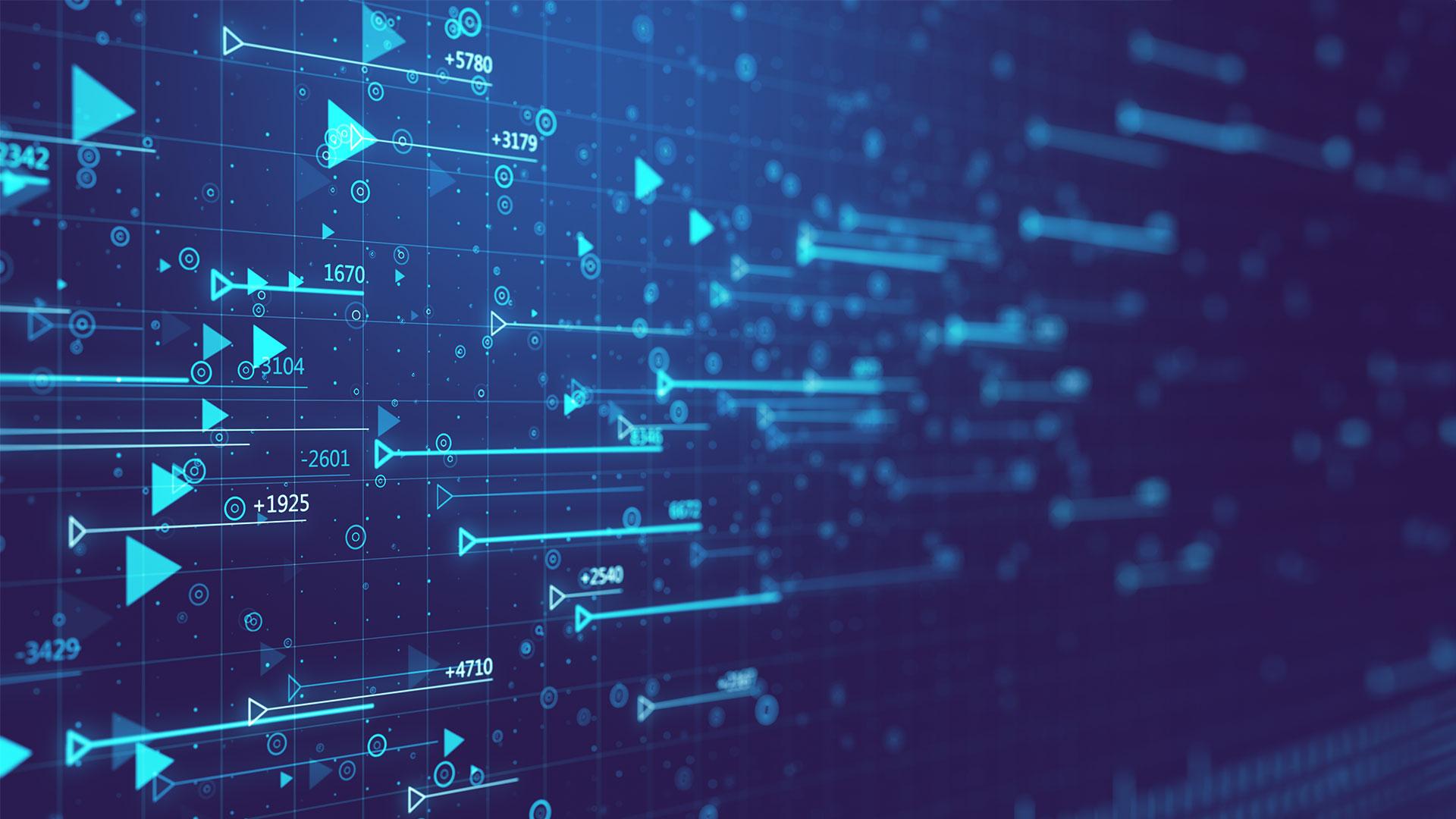
At the end of November 2025, the new language format ISO 20022 will be the only acceptable standard for cross-border payment instructions and reporting messages – SWIFT MT (message type) 103 and 202COV messages will be replaced with MX (message exchange) pacs 008 and 009
Past the minimum requirement to update back office systems to be able to process MX payments, there are divergent views on how much to invest in making this data accessible to downstream systems and upgrading them to use it. In a year of multiple other regulatory deadlines, macroeconomic and geopolitical headwinds to profitability, there is little room for “nice to have” regulatory expenditure. But banks also recognize the importance of getting data ready for AI – the efficiency and effectiveness opportunities are immense across every process.
So, is processing MX data a regulatory tick-box exercise or should it be part of AI strategic investment? How much could it improve your correspondent banking detection outcomes at a reduced long-term cost?
What are some of the potential costs to correspondent banks?
Development costs
A significant investment is made to parse and cleanse the unstructured data fields in MT messages to improve the quality of name and address information feeding into Entity Resolution.
Data quality monitoring costs
Huge volumes of payments can fail data quality checks, requiring impact analysis and management time to risk accept the findings.
Operations costs
There is a direct link between data quality issues in the payment data and the volume of alerts requiring investigation. Correspondent Banking transaction monitoring can be badly affected due to sole reliance on transaction data to identify the parties. Even a fuzzier level of entity resolution to resolve transactions with slight variances in name/address data can leave even the best technology solutions vulnerable to overlinking, where the data quality or completeness is poor.
What are the opportunities with MX data?
AI-ready data
MX data exponentially increases a model’s capability to deliver better outcomes at reduced cost because MX data elevates the quality of input and makes it more accessible to a model, leading to more accurate, enriched entities. These entities can be monitored more effectively, meaning better risk detection with fewer false positives.
Know the agents: Structured fields for all banks
Every bank in the payment chain has a dedicated field for its position. Benefits include easier identification of transactions involving nested banks and extraction of country code from the structured BICs to cross-reference with the transaction address data to identify non-resident parties.
Know the parties: Structured name, address, and country fields
Clear delineation of detailed data points like flat numbers, house numbers, and cities makes it significantly easier for solution providers like Quantexa to use these to mitigate overlinking and underlinking, leading to fewer, better alerts.
Improving country identification is also essential for entity resolution as well as for scoring High Risk Jurisdictions.
Know the ultimate parties: Ultimate debitor and creditor fields
Identify payment-on-behalf situations where the payer is distinct from the receiver of the invoice and initiates payment from their account, and/or a payment is credited to an account, but which may be on behalf of the true ultimate creditor.
Know the purpose and the regulatory perspective on the payment: Structured remittance information
Monitor for suspicious activity is not black and white like regulatory reporting, but “category purpose” could be a useful indicator of lower risk payments and used to de-prioritise their monitoring.
How to maximize opportunities from the transition to MX data
This new data is too good to overlook, so how should you proceed? The cost and challenge of your road ahead depend on your solution technology. Re-platforming in-house systems to add and extend existing fields can be costly, time-consuming, and the expertise to execute may be lacking. In short, there has never been a better time to invest in new technology.
The best return on investment lies in getting data right first. Quantexa's Decision Intelligence Platform specializes in unifying, enriching, and contextualizing data, which powers effective detection outcomes.
Since our inception, Quantexa has demonstrated a leading capability to unite disparate sources where the same data points are not consistently populated but refer to the same real-world entity. Our platform can consume any fields parsed in your data with no character limit issues, and integrate these new fields into existing capabilities seamlessly.
The Quantexa platform provides you with a contextual fabric to put your connected data into context. The contextual fabric is essential to fuel any AI aspirations. The more complex the risk you are trying to detect, the stronger your trusted data foundation needs to be. Quantexa’s contextual fabric helps unify the different data points to have a common picture, and our knowledge graphs create and visualize the network. Quantexa's knowledge graph capabilities aren’t like a generic graph database, they are a means to an end in our Decision Intelligence platform.
Tier 1 banks globally trust our Decision Intelligence Platform to tackle complex challenges of pseudo-customer and counterparty identification with MT data, and the possibilities are greater with MX.
With MX data, the opportunities for meaningful graph connections increase, which are consumable by our AI models to power you to even better risk detection outcomes. Investing in MX data does not have to be at the expense of your AI ambitions, but shortcutting the data step will set you back.
To find out more about how Quantexa can maximize the opportunities of MX data, contact us today.
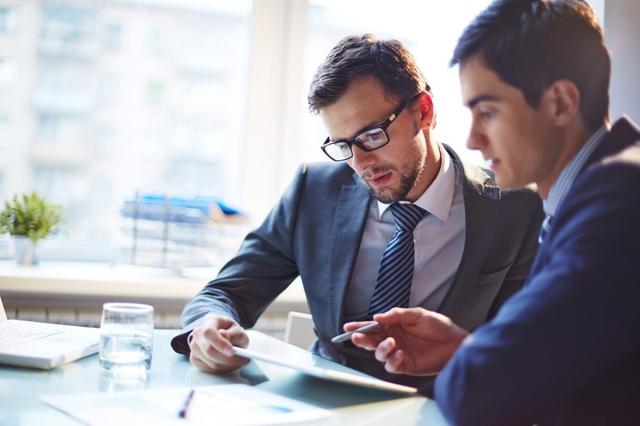