Are You Suffering With the Challenges of Defensive Alerting?
It could be time for a new contextual approach to monitoring.
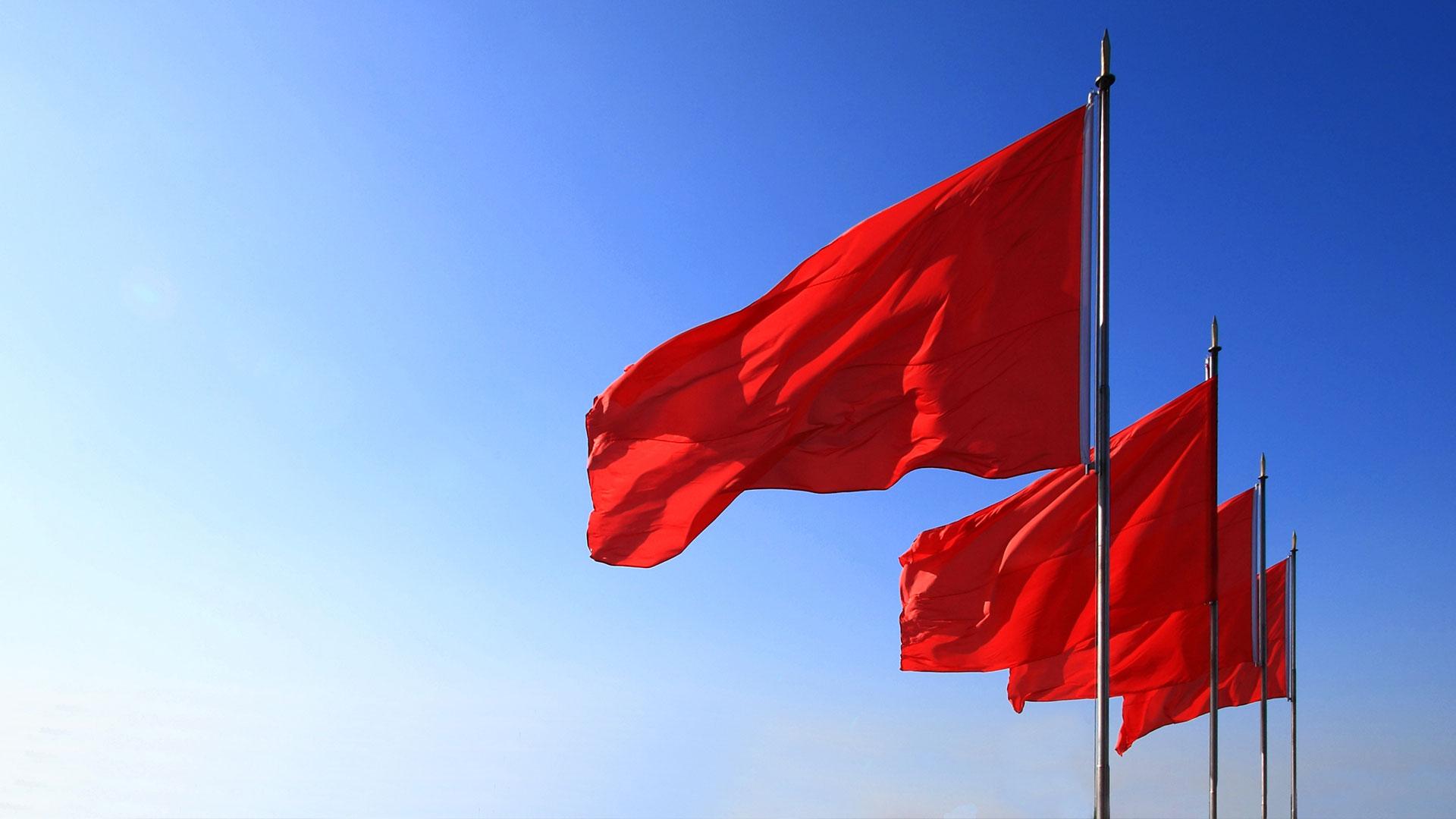
Banks spend a large amount of time mapping regulator-provided red flags to detection rules/scenarios, often on a one-to-one basis. Every time a regulator or industry body releases a new set of red flags or indicators, a new rule or scenario may be introduced.
While this approach is well-intended, it has resulted in the reactive concept of defensive alerting and, in turn, defensive AML reporting – something which regulators are increasingly highlighting as problematic and time-consuming.
What is defensive alerting?
Defensive alerting defines the process of producing high volumes of low-quality false positive alerts, usually set against a singular red flag. While in combination red flags have immense value to detect risky or anomalous activity, in isolation, they can be less useful.
As a result, this has seen the growth of operational triage teams dealing primarily in the rapid clearance of low-quality alerts at a transactional level, without considering the context of the transaction against a customer’s usual activity or the activity of its peers. For instance, a transaction-focused alert closed as a false positive by a human analyst within five minutes is likely to be a meaningless exercise. However, the presence of multiple risks on a single network of customers and/or counterparties is a greater indicator of risk.
Are you suffering from defensive alerting?
Unmanageable numbers of unique detection rules/scenarios, perpetually high alert volumes, short investigation SLAs, and low alert-to-regulatory filling conversion rates are all symptoms of a sub-optimal approach to Anti Money Laundering (AML) transaction monitoring (TM) that is overly focused on rules-based detection.
The limitations of defensive alerting
A defensive alerting approach inadvertently reduces the effectiveness of a bank’s controls due to the “needle in a haystack” approach of alert reviews. Balancing untenable alert volumes against timeframe requirements, both operational and regulatory, means a compromise on quality investigative analysis, thereby introducing risk.
Past attempts to solve this problem have focused on treating the symptoms, including:
Tuning of alerting parameters and thresholds
Segmentation of customer base and alerting at a customer level (I.e., creating a smaller haystack)
Large-scale investigations teams and low resource retention rates
Applying automation to alert triage, and data gathering activities.
The knock-on effects of defensive alerting are wide-reaching and even impact the ability to retain top talent. Analysts who were originally sold on the premise of fighting financial crime, quickly become disillusioned with their place in process-driven operations – this risks losing the motivated and skilled investigative talent that will be required to detect and uncover complex and often hidden risks, such as those seen in the Russian Laundromat.
However, a manageable and risk-focused strategic approach requires a fundamental change intact. It demands an approach that automates many of the repetitive manual checks, considers the entirety of the potential risk and suspicious activity from multiple internal and external data angles, and importantly, examines and assesses each risk attribute collectively – in other words focusing on context.
Introducing a contextual approach
Contextual Monitoring is a fresh ‘investigate to detect’ process and signals a more effective means of tackling financial crime and fraud. The Financial Action Task Force (FATF) defines Contextual Monitoring as:
“The ability to join and connect together data from different systems and sources to create context and meaning to identify significant connections and improve accuracy. It employs advanced algorithms which allow more sophisticated scoring and analytical approaches.”
Contextual Monitoring is powered by Entity Resolution, the process of dynamically connecting and enriching internal and external data to reveal a single view of entities across an organization, and network generation, which maps out relationships to identify new, previously hidden risks faster and more accurately. It brings a shift in focus from alerting on singular transactional red flags to combining multiple risk indicators on a single network, fed into predictive analytics, and followed up by highly skilled investigative staff.
Leveraging machine learning triage to manage alert volumes
Machine Learning and Artificial Intelligence (AI) play key roles in the fight against financial crime – and in a Contextual Monitoring approach. However, there has been an over-focus on the use of predictive analytics within auto-triage models to cover up deficiencies in transaction monitoring.
Triage models themselves can be overly reliant on static data points as features and often replicate rules-based decisioning, creating a misconception of successfully replacing human investigations. However, when you have >95% false-positive alerts, it is not difficult for AI to look as though it is successful at closing alerts.
An approach like Contextual Monitoring would enable data scientists to focus their attention upstream by creating more effective and efficient strategies through the detection of truly anomalous risky behavior.
How to uncover the next Russian Laundromat using Contextual Monitoring
Do we really expect to uncover the next Russian Laundromat with an out-of-the-box rule based on a single risk data point or red flag?
Taking the Russian Laundromat as an example – those involved were not identified through a single red flag – but through the combined presence of shell companies, the rapid movement of funds and luxury goods, high-risk industries, and suspicious networks.
The process of enriching internal data with external sources and building networks of relationships to identify new, previously hidden risks enhances detection with models that leverage network-based context, which in turn reduces false positives and generates more accurate alerts.
To truly succeed in the fight against financial crime, financial institutions must combine multiple risk indicators on a single network, enabling highly-skilled and empowered teams to investigate genuine risk alerts – and a Contextual Monitoring approach is proving to be the most effective solution.
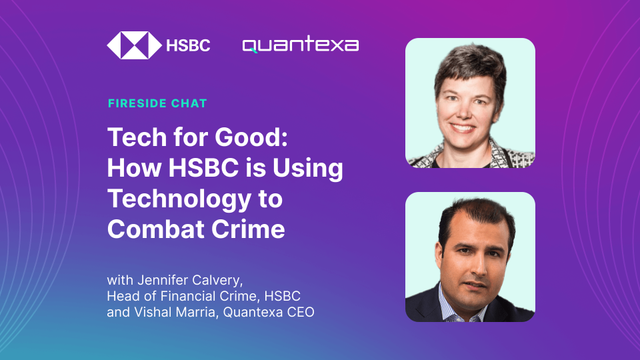