How to Harness Contextual Insights Into Financial Markets Surveillance
Using data integration, graph analytics, and advanced analytics, financial institutions can improve the detection of sophisticated criminal collusion.
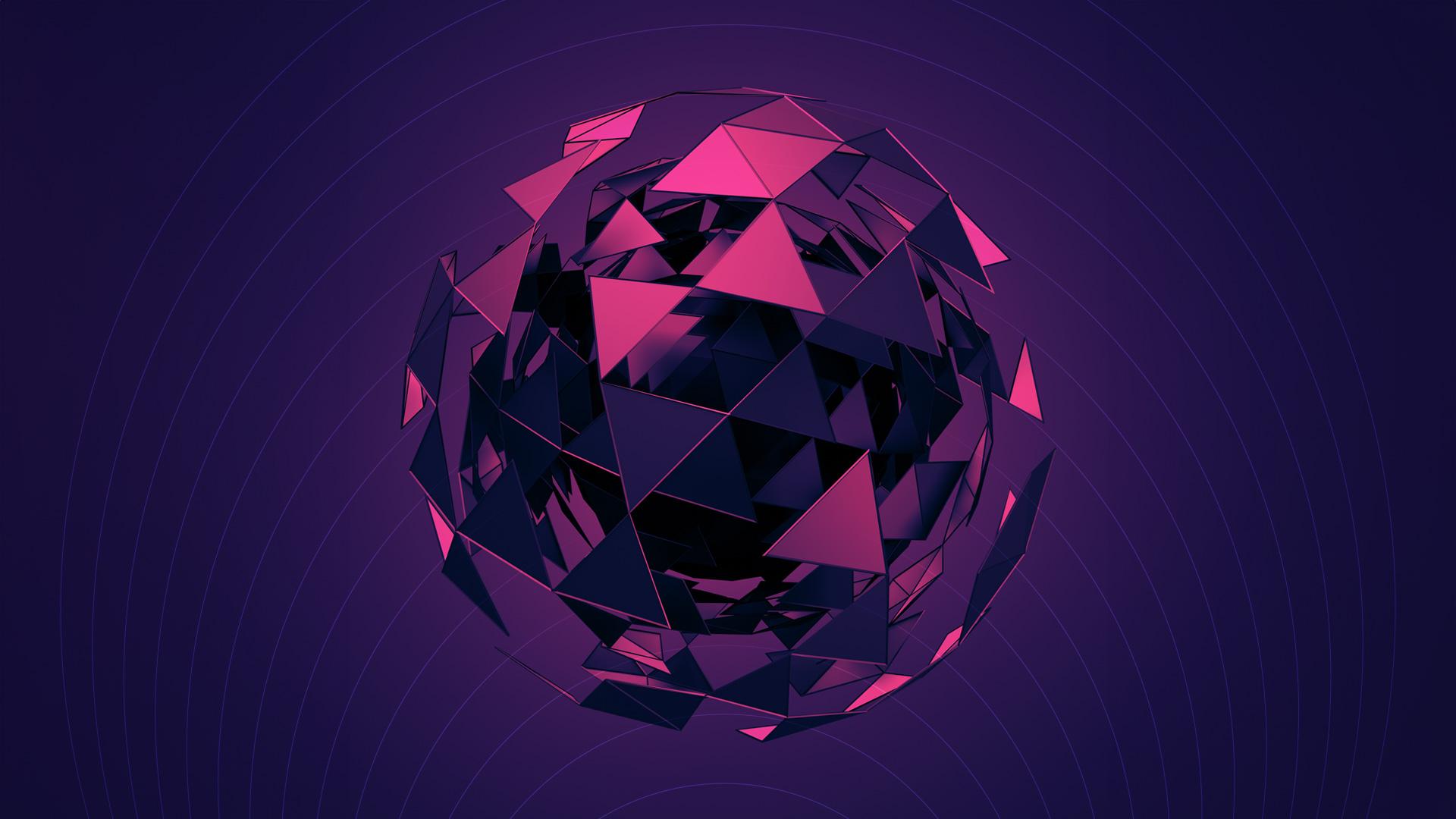
In recent years, financial markets have witnessed a surge in market conduct cases, ranging from insider trading and unauthorized trading to complex market manipulation schemes. Surveillance systems have not kept pace with criminals. As regulators intensify their scrutiny of market participants, the need to embed context into surveillance mechanisms has never been greater.
In this article, we highlight the contextual approach to surveillance and how it can help organizations to bolster their surveillance framework.
What is the contextual approach?
A contextual approach to surveillance involves analyzing trading activity and market events within the broader context of relationships, market conditions, and trader and customer behavior. Rather than relying solely on individual trades, isolated data points, or siloed monitoring, the contextual approach takes a holistic view of activity, utilizing data from disparate internal and external data sources to provide insights into the underlying factors and potential motivations for trading decisions.
Why context is key
Traditionally, financial market organizations have used multiple siloed surveillance systems, such as Trade, E-Comm, AML, Unauthorized Trading, Personal Account Dealing, and others, to supervise various aspects of trading. However, when working in isolation, these systems are unable to build a holistic view of trading activity and lack the contextual understanding to differentiate between legitimate trading behaviors and market misconduct.
In addition to providing a holistic view, a contextual approach (that uses network analytics) can provide valuable insights into uncovering collusion, which is often at the heart of market misconduct behaviors. Collusion typically involves sophisticated schemes orchestrated by multiple parties across different jurisdictions and trading venues. These schemes involve subtle coordination, indirect communication, and carefully disguised trading patterns.
Take, for example, spoofing activity, which has been a hot topic in the industry for the last few years. Spoofing is a deceptive trading strategy in which a trader or a customer places orders on one side of the order book, with the intent to cancel the order and execute on the opposite side. Traditional surveillance systems will look for a single trader/book or a customer executing this strategy—but what if two socially connected customers collude to do this, and are on opposite sides of the order book?
Similarly, for detection scenarios that look at price movement as an initial trigger, checking whether the price movement was consistent with the sector can reduce false positives significantly. In addition to finding more risk and reducing false positives, context helps investigators make better decisions. All the information relevant to the detected anomaly is presented to the end user in one place. This makes their investigation process more efficient.
Organizations are already benefiting from this holistic approach in their AML programs. Now, it’s time for surveillance teams to take the same leap to become more effective.
How to embed context in surveillance
Data integration: Integrating diverse data sources—including trading records, outputs from siloed surveillance systems such as Trade Surveillance and E-Comms, news feeds, market data, and corporate registry data—into a centralized platform provides a comprehensive view of market conditions, trading activities, and relationships between the trading parties. This holistic approach enables better risk detection and reveals legitimate business reasons behind certain transactions, thereby reducing false positives.
Network visualization: By analyzing the relationships and interactions among the traders, customers, and other entities, a contextual approach can uncover clusters of customers, traders, and entities engaged in potentially collusive illicit activities. It enables the visualization and analysis of relationships, interactions, and transactions within the financial ecosystem, empowering surveillance teams to make better decisions and detect collusive activities.
Advanced analytics: Techniques such as pattern recognition, sentiment analysis, and machine learning models can uncover subtle patterns and anomalies indicative of coordinated activity among market participants, significantly enhancing the detection of collusion in trade surveillance.
As financial markets continue to evolve and criminals become more sophisticated, the importance of moving to a contextual surveillance approach cannot be overstated. By harnessing the power of advanced analytics and context, financial institutions can revolutionize their surveillance capabilities, enhancing detection accuracy and safeguarding market integrity for the benefit of investors and stakeholders alike.
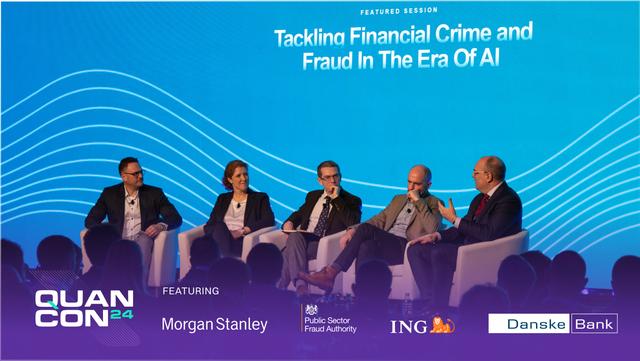