Generating a Holistic View of Legal Hierarchies Using Context
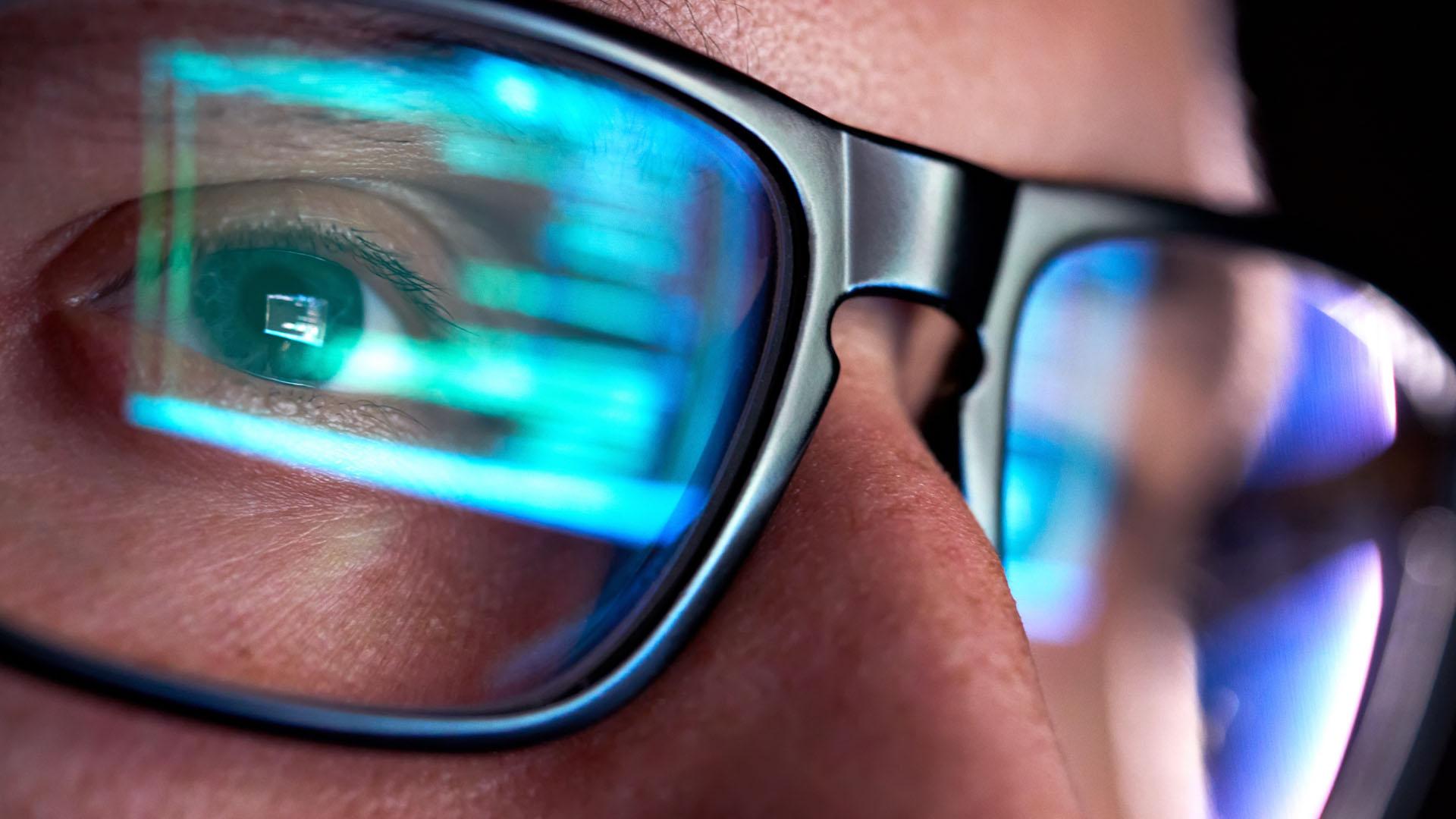
In recent years, regulators have tightened their requirements for tackling money laundering and terrorist financing. Fraudsters often use offshore accounts and complex corporate structures to circumvent the law and mask their criminal activities. But to outpace bad actors organizations need to better understand the network surrounding suspicious business activity.
The challenge legal hierarchies pose for businesses
Identification of Ultimate Beneficiary Ownership (UBO) is a crucial element in several applications, including KYC and Credit Risk, as well as finding financial crime. There is no universal definition of UBO and exploring corporate structures in different ways can give varying results for various use cases. Being able to identify the UBO drives compliance to regulatory requirements, and better risk assessment of the business in question.
Defining a single view of complex, corporate structures
Defining a single, holistic, and comprehensive view of clients with complex structures can be a challenge. Banks often have multiple internal views of corporate hierarchies that are used alongside external data sources. So, how do you merge this data to create a unique view of a client legal hierarchy? And how do you identify the UBO in such structures?
The latter might be easy when you deal with sole traders, but how can organizations identify the person or group of persons that hold the power in complicated structures, such as complex companies. You might end up in a long chain of businesses and individuals where it’s easy to get lost in the search for the UBO.
To tackle this problem, you first need to have a comprehensive view of each of the businesses constituting the legal hierarchy. This can be achieved by leveraging innovative AI and Machine Learning (ML) technologies powered by entity resolution and network generation.
Entity Resolution allows you to bring information on businesses together into one single data point and generates a likelihood to determine which identities are a match and which are not. This is an essential step in building a single view of your data and connecting information from different sources about the businesses being analyzed.
Network generation connects business entities based on their ownership structure (among other factors). This also allows you to discover hidden links which can be challenging when looking at data in isolation.
Once you have a clean view of a client’s network with resolved entities, the context offered by the network can enable advanced network analytics which can be used to extract new relationships and identify key entities in the network.
Applying network analytics to extract insights
Network analytics uses relationships represented in the network to uncover new insights. There are several network analytics algorithms, starting from simple heuristics to deep learning methods that can be used to extract information from a network.
In the context of a legal hierarchy, these algorithms can help to extract relevant structural properties, such as:
The number of businesses and individuals of different sizes in a legal hierarchy
Whether the legal hierarchy contains cycles (and hence risky ownership patterns)
Which nodes have most control over the network among other characteristics.
Cycle detection in practice
Cycles in corporate ownership can occur naturally in complex investment portfolios, or deliberately when efforts are being made to obscure the true ownership. While relatively rare, this pattern can cause naive algorithms to fail and return null results.
Cycle detection: One common method to detect cycles is to find ‘strongly connected components’, which imply that a cycle must exist somewhere in the network. This is very helpful in a legal hierarchy problem as shown below:
A owns B, B owns C, C owns A, Mr X owns C. In this case we can safely below assert that Mr X also has an element of jurisdiction over A and B.
Further investigation, including the use of machine learning, could identify Mr X to be the UBO or have some other more significant relationship to all businesses in the hierarchy.
How link imputation can reveal further risk
The UK has relatively high-quality ownership data, even of small businesses. But this is not the case globally. There are exceptions to this even in the UK such as Scottish LLPs. Bad actors are well aware of these weaknesses and have, in the past, made use of them.
The data generated through network analytics, along with the other classical (tabular) features, can be used to impute links by applying advanced Machine Learning algorithms. In a legal hierarchy domain you can add links in a corporate structure by imputing ownership and potentially finding new UBOs.
Representing data via networks starts with context
There are many techniques that can help organizations explore and extract useful insights from complex legal hierarchies. These include better risk assessment of businesses that are unusually complex compared to competitors. (For example a “young” SME (Small Medium Enterprise) that has multiple hierarchy layers or a mid-market sized company with many shell companies and links to low-tax jurisdictions).
The power of representing data via networks lies in the fact that even differing networks can have similar structural properties, which is why using graph analytical approaches yield big business value. To start, you just need the right context.
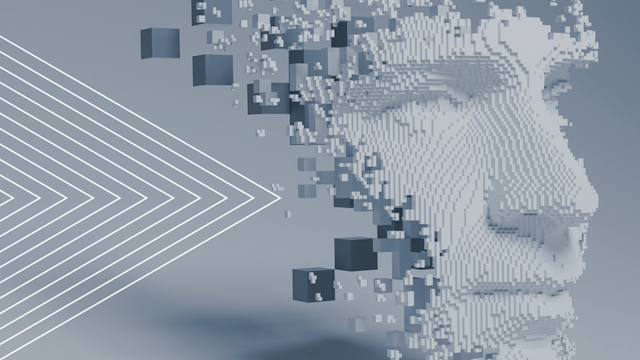