How to Distinguish Individuals From Businesses In Your Data
A key step in understanding the relationships between businesses and individuals is first identifying which is which.
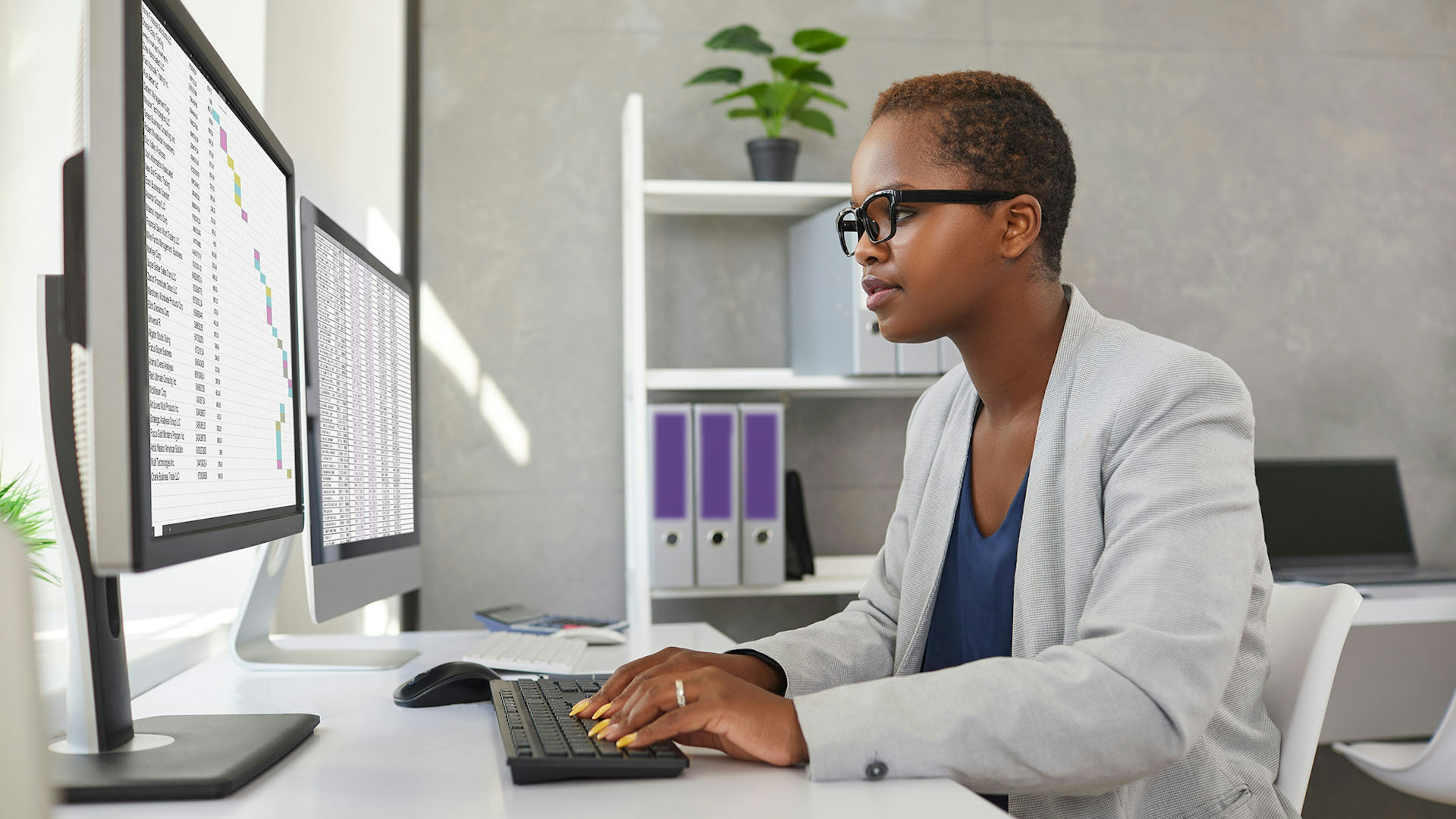
A first step in understanding the relationships between businesses and individuals is identifying which is which. It would be understandable to think that this would be a straightforward task – how could “Quantexa” be anything but a business? However, if you consider the John Lewis-es and the Peter Jones-es of this world, you begin to see how this problem might be more nuanced than it first seems.
A bespoke machine learning solution is the answer to tackling this problem on a global scale. But the key to success is not only reliant on the machine learning, but on the data that is fed into it. Creating a varied and representative list of names and businesses, and providing relevant additional “context” to each, is essential. Outlined below are the five factors which have contributed to the creation of this new model which better discriminates between individuals and businesses as part of an advanced Entity Resolution:
Context is everything
Machine learning algorithms perform best when they are given as much information as possible. If you can tell the algorithm extra information over and above the name you are trying to identify, then you’ll gain a big advantage.
Take, for example, trying to classify the name ‘John Lewis’. It could be the UK-based department store, or it could be just a name. If you knew this entity was based in the US, you would quite comfortably be able to rule out the department store and feel certain it was an individual.
It’s fundamental that this model works across various countries and languages, so providing the algorithm with as many reference points as possible is vital. If businesses in a particular region follow a specific naming convention, then you can give the training algorithm a head start in its decision-making process.
Acquiring a broad variety of data
Both business names and individual names can appear in many forms. Take the name ‘John Andrew Lewis’ – he may appear as J.A. Lewis; John Lewis; Lewis, John Andrew and so on. Similarly, businesses can appear as their full legal name (e.g., Quantexa Limited) or by a heavily abbreviated version (e.g., Quantexa, or even Q). It is essential that the algorithm can learn from a full range of different names and formats.
Imitating abbreviations and misspellings
Although a range of data sources (both open and licensed) of well-structured individual and business names exist, it is more difficult to find labeled sources that better reflect user-input names. When looking to parse a Swift message, for example, you can reasonably expect a business name to be misspelled or abbreviated.
The data source in use for training includes samples of data where the names from the ‘golden sources’ have been artificially misspelled in such a way to mimic the way humans tend to misspell words. Similarly, artificially abbreviated forms of the names are included to replicate names entered in shorthand messages, and even blog and news posts.
Building a relevant machine learning model
Building a model which can incorporate contextual information will result in a significant performance boost. Of course, there are some cases that will never require being seen by a model – for instance, our analysis shows that there is no known individual with the word ‘and partners’ in their name. Focus can therefore be turned to the less straightforward cases.
Quantexa’s model is a neural network consisting of Long Short-Term Memory (LSTM) cells to handle the raw text, combined with fully connected layers to build in the additional context (which is included in the form of straightforward tabular data with details such as the country of registration/residence.) When the two layers are combined, it provides an overall decision.
It looks something like this:
Going international
It is common for different countries to have varying business name formats (Think “Inc.” in the US and “LTD” in the UK).
But how do you overcome the challenge of identifying a business that’s been named after a particular individual – which is a common practice for family-run restaurants in smaller countries?
In these instances, it might be necessary to bring in a native language speaker to help assist the entity resolution process. Combining human intelligence with machine intelligence will help the Quantexa model distinguish between entities across all cases.
Combining human intelligence with machine intelligence will help the Quantexa model distinguish between entities across all cases.
Overcome ambiguous classification with a machine learning approach
Using a contextual machine learning approach has given Quantexa substantial uplift compared to rules-based methods, in some cases increasing accuracy by over 40%.
The machine learning approach also gives significantly more flexibility to the user by outputting a probability rather than a hard decision, meaning ambiguous cases (such as John Lewis) can be appropriately handled for the business problem being addressed. It is particularly important to consider the cost of misclassification for businesses and individuals. Frequently, such classification and disambiguation occur before matching to other data elements.
So, the question is – are your matching algorithms sophisticated enough to handle these ambiguous classifications?
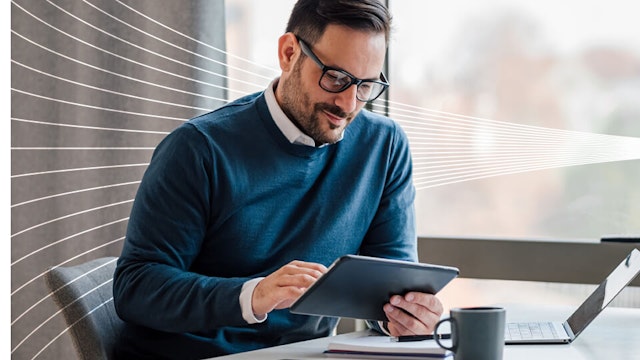