Our Decision Intelligence Platform
Turn ambiguous, siloed data into the most trusted, reusable resource for your organization.
Learn more about the Quantexa Decision Intelligence Platform
The platform to transform your decision-making
Open and modular for outcome-driven solutions
Integrate any source at scale
Accelerate your data onboarding with low-code, scalable, high-performance data preparation and ingestion.
Connect all internal and external data at scale with unprecedented accuracy for multiple use cases.
Improve data quality as part of the unification process.
Harness the power of AI
Our AI is flexible, explainable, and adaptable. It allows us to address a wider set of business problems and deliver more powerful solutions for our customers.
We combine a wide range of different approaches, techniques, models, and algorithms across our DI platform.
We believe in the power of human and AI teaming for better augmented decision-making, enabling our customers to address a wider range of use cases with greater confidence.
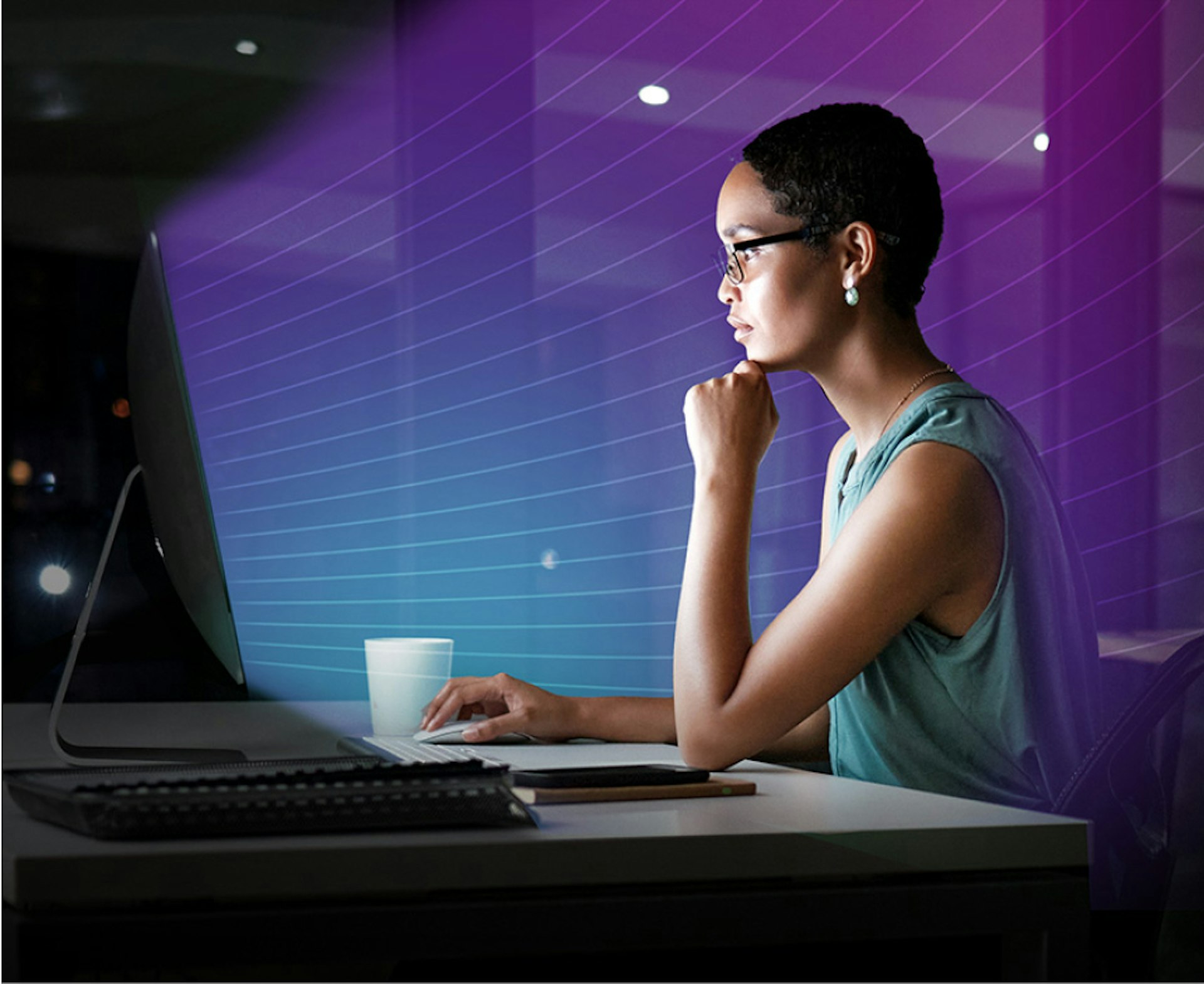
What makes our platform different
What you can expect
60 billion
Records managed with enterprise scalability
99%
Entity Resolution accuracy
75%
Reduction in false-positive alerts
20%
Proven de-duplication of records
Solve it with Quantexa
Data Management
Build a trusted data foundation to deliver context for decision-making.
Learn MoreCustomer Intelligence
Enhance customer experience and accelerate revenue growth with a 360-degree connected view of customers.
Learn MoreKnow Your Customer
Detect risk in real time to identify unknown risks and deliver more accurate risk ratings.
Learn MoreRisk Management
Revolutionize enterprise risk assessment with a holistic understanding of borrowers, their counterparties and relationships.
Learn MoreFraud and Security
Uncover hidden fraud risks with a contextual approach to detection and prevention.
Learn MoreAML and Investigations
Reduce false positives and focus on real risk by modernizing your AML monitoring, detection, and investigations.
Learn MoreYou don’t know the risks you can’t see
Explore Quantexa’s financial crime solutions to see our unified approach to combating economic crime, and fraud.
Who we help
Banking
Banking
Insurance
Insurance
Telecommunications
Telecommunications
Health and Social Care
Health and Social Care
Government
Government
our ecosystem
More than a platform
Our world-class ecosystem and growing user community are here to support your ongoing success.
Quantexa Partner Ecosystem
Our partner ecosystem represents some of the best and brightest companies across the globe.
Quantexa Community
A place for customers and partners to learn, share, and collaborate.
Customer Services
Maximize your investment in Quantexa through our Expert Services, Learning Academy, and support.
get in touch
See it in action
Protect, optimize, and grow your organization with Decision Intelligence. More is possible with the right data in the right context.
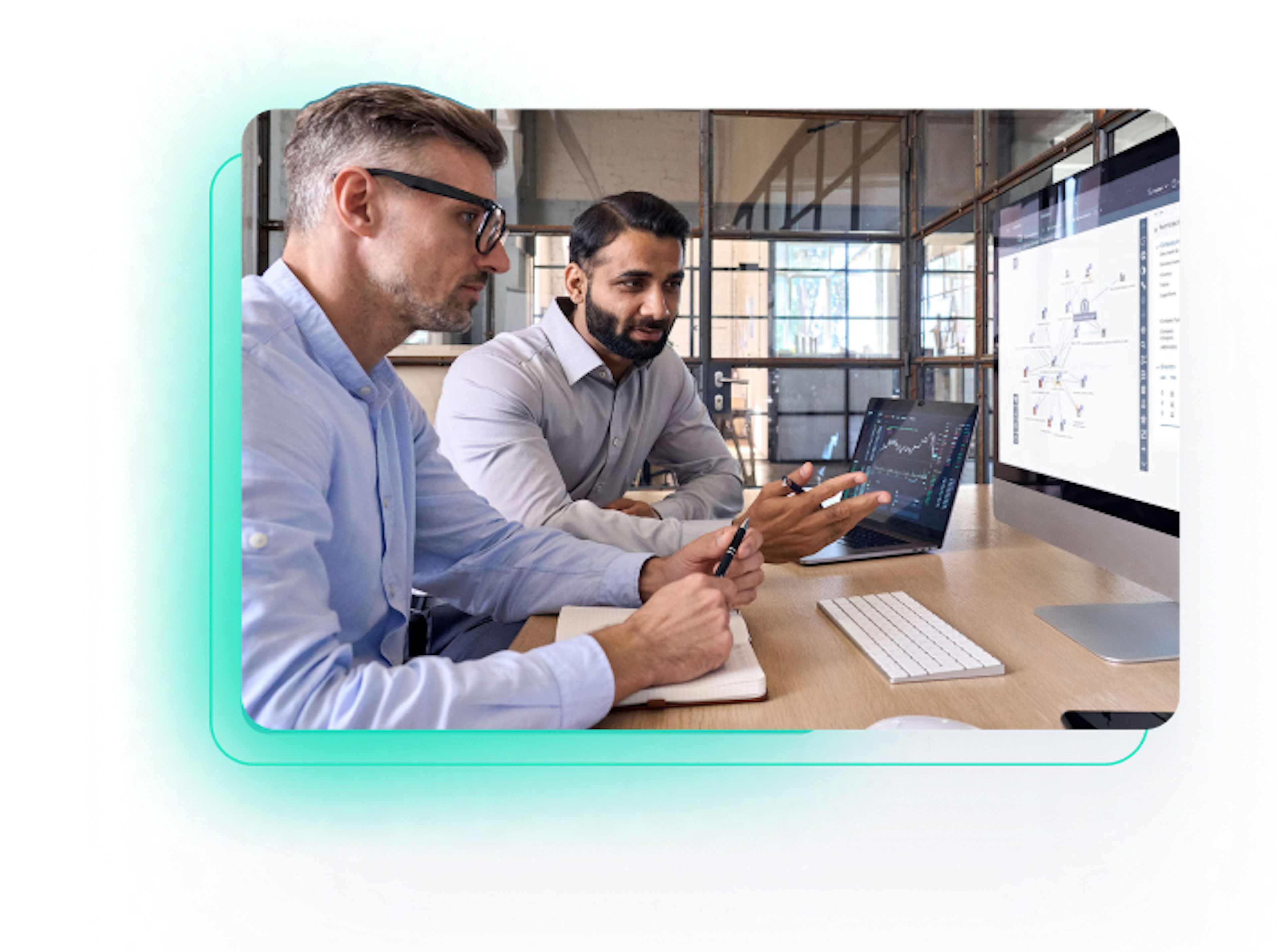