How to Build Additional Context Into Your Machine Learning Algorithm
Introducing network features can help your organization detect risks at speed.
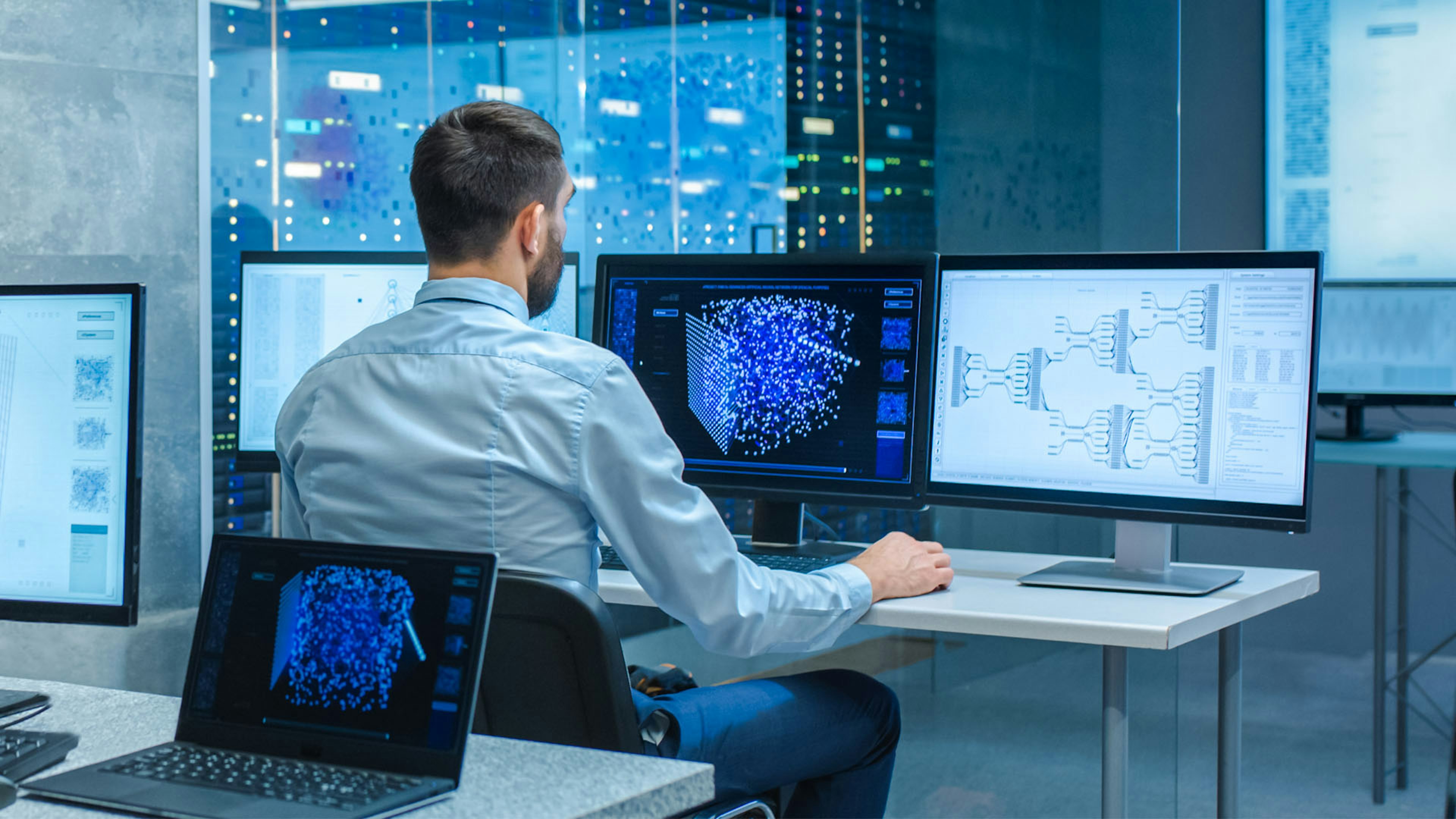
From detecting traffic obstacles for self-driving cars to recommending products, machine learning (ML) plays a key role in most organizations’ decision-making processes. For financial services organizations, machine learning is increasingly critical in identifying risk, within consumer and corporate entities.
The importance of context
In the world of risk modeling, the input data points (or features) are particularly important – usually even more important than the choice of model or algorithm. In an industry with significant regulatory pressure for transparency and explainability in modeling, model choice is often restricted meaning the choice of input features can be the core reason for success (or failure) of the model. So, the key question is – how can we bring as much context into our features as possible?
Network-based features provide an excellent way to inject a huge amount of information into your model, whilst keeping the required transparency and explainability One way of achieving this is by using bespoke document-entity networks to generate features describing how businesses and individuals are related to each other. At Quantexa, we’ve used network features describing relationships between companies and their directors as key inputs into our ML Shell Company detection model, which has resulted in a 20% uplift in performance compared to using just record-level features.
The model output – predicted shell companies and the agents creating them – has a range of applications for enhanced risk detection across AML (Anti-Money-Laundering), KYC (Know-Your-Customer), Risk and Fraud.
Introducing network features
Networks can be used to model relationships between entities in many different circumstances. For example, networks of payments between parties can be used to find signs of financial crime. Searching for certain shapes in the network (e.g. cycles of similar sized payments) can lead to the detection of risks that would not have been identifiable by looking at transactions in isolation. If a list of known instances of financial crime is available, then network features such as the number of U-turn or cyclic payments can be used as part of a supervised learning model to enhance the prediction of future risks.
One obvious network to use as a basis for modeling corporate risk is an organization’s legal hierarchy which includes its directors, shareholders, and subsidiaries. Simple characteristics such as the size of this network, the number (or density) of connections, and the number of layers can all be useful dimensions for segmentation or feature generation for supervised learning models.
The power of networks in machine learning
In the Quantexa ML Shell Company model, we have used a combination of features derived from organizations’ wider networks (including their legal hierarchies and director networks) and from company registry records. These network features include the identification of network shapes and patterns, as well as using techniques from graph analytics (including centrality analysis) to create new information about the entities in the network, like finding directors with high importance in the network.
The director in the network has control of four organizations, all with single shareholders and based in profligate (or highly connected) addresses. Creating features such as ‘director’s company is based at a profligate address’ and ‘director shares directorship with a 100% shareholder’ can be very informative in finding shell companies. In addition, the director above has high ‘page rank’ (centrality) in the wider network.
These new network characteristics are used as features within a company-level model. This evaluates if each company is likely to be a shell based not just on its own characteristics (size etc.) but also on this network context.
Including network features in the model significantly enhanced its performance and has so far led to the identification of over ten thousand new shell companies not identifiable from record-level models alone. The use of network features to provide context has added significant value in other areas too, including scorecard tuning, and even data quality analysis.
Unearthing more complex patterns
Recent research has uncovered a plethora of techniques for using networks as part of a machine learning lifecycle. In particular, the field of Graph Learning has evolved to use networks directly in machine learning algorithms (without creating summary features from the network). This will enable more complex patterns to be found in the networks which correlate with risk.
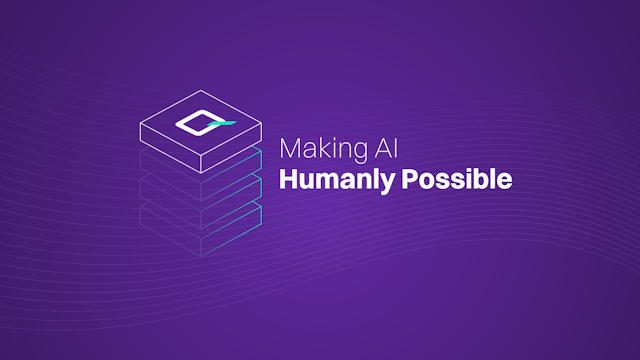