6 Data Readiness Challenges for AI Deployment – And How to Solve Them
Data readiness is critical for successful AI deployment. Find out how to overcome the six most common data challenges.
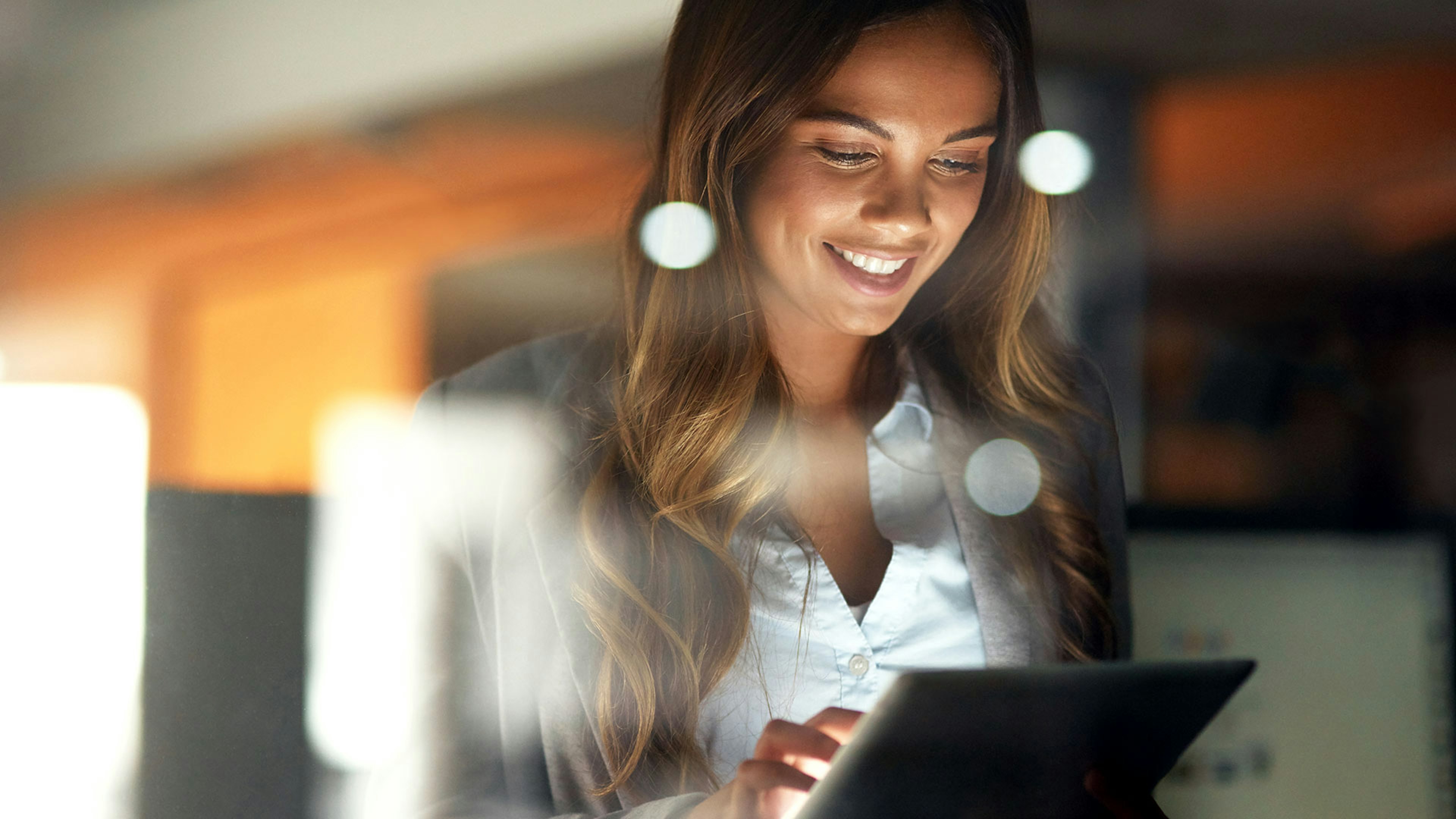
The rate of artificial intelligence (AI) is growing for financial services companies. In a 2021 NVIDIA survey, 83 percent of financial services professionals who responded agreed that AI is essential for their company’s success. In fact, in the February 2022 State of AI in Financial Services report by AI Forum, over 61 percent of financial professionals said they already use or plan to use AI for decision-making.
This shift to AI requires moving from big data to quality data, a concept called data readiness. Data readiness, along with a mix of the right technology and talent, is critical for the successful deployment of common AI use cases across financial services companies.
As you consider AI to help drive your organization’s future, understand why you need to be data-ready, and what that means for AI. In this post, discover what data readiness is, why it’s important for AI, and the six data readiness capabilities you need for successful AI deployment.
What is data readiness?
The journey to AI for financial services begins with data readiness. Data readiness refers to all the necessary steps to ensure your data is prepared for AI purposes. It considers the foundational, operational, and transformational quality of your data to enable successful AI deployment:
Foundation: Your data has the appropriate infrastructure and interfaces.
Operation: Your data is suitable for management and governance to maintain your AI solutions.
Transformation: Your data is optimized to ensure success with your AI deployment.
Data readiness comes in three levels, which start at Band C and progress to Band A:
Band A: Refers to contextual data and its appropriateness to answer a specific question or serve a particular analysis.
Band B: Refers to the validity of data — its accuracy and completeness to define a context.
Band C: Refers to the accessibility of the data, and whether it’s ready for analysis software.
The success of your AI is only as good as the quality of your data. Therefore, you need to understand your current level of data readiness, so you know what work lies ahead for you to prepare it for AI purposes.
Why data readiness is critical to AI
AI relies on data readiness for several reasons. The first reason is to gain some control over the vast amounts of unstructured data, such as in news stories, articles, intelligence case files, and emails, which account for 80 to 90 percent of all data. The value of that data is a gold mine of information and insights that no amount of research could ever provide. According to an article from the MIT Sloan School of Management, the retail and finance industries are leading the way in using AI to capitalize on this data. For financial services companies that are considering AI deployments, they must invest in ways to ensure their unstructured data is ready to help drive their future.
The second reason for data readiness is to allow companies to deliver personalized service. In 2021, Google announced that, by 2023, it would stop supporting third-party cookies, which are used to personalize experiences across the web. Soon companies must use their own data and websites to create personalized experiences for their customers. Data readiness will be critical to enabling intelligent decision-making to drive that level of customization.
Data readiness for AI
6 data readiness capabilities for successful AI deployment
For financial services use cases, industry professionals highlighted six primary capabilities necessary for data readiness.
1. Data lakes
According to the AI Forum survey, 19 percent of banking and financial services organizations use data lakes to connect to and process vast quantities of data in a large cluster. The data comes from multiple source systems, organizations, and even third parties. Eventually, data lakes become a dumping ground of raw data, making it difficult to aggregate or operationalize it. As a result, data scientists and engineers must tediously sort through the data to make sense of it.
To prepare for AI deployments, these organizations need to combine their data sources within the data lake to create a single view of their records. By combining this data, AI platforms can analyze a previously prepared single view and create insights to help guide the organization.
2. Network of relationships in the data
Another challenge of data readiness is the ability to break through the segregated data of organizational silos. In these silos, each department operates according to a narrowed view of its customers, with no visibility into or clarity of the broader relationships that exist across the organization. In the AI Forum survey, 19 percent of respondents indicated a need to build a network of data relationships.
Breaking down organizational silos to build a network of data relationships starts with network generation to create a single customer view based on quality, connected data. Only then can companies see how a client is engaged across various departments, and how multiple clients might be related. They can also see which clients in one department can provide referrals to win new business in another department.
3. Analysis of text and unstructured data
Of the AI Forum survey respondents, 17 percent indicated issues with the ability to analyze text and unstructured data to create context. Contextualizing unstructured data can occur through Natural Language Processing (NLP) to extract entities across all data sources. Or it can involve the labor-intensive, painstaking process of manually searching the unstructured data for a set of known entities.
Preparing vast amounts of unstructured data for AI deployment requires applying contextual search. This approach helps financial services companies find the most relevant information in their unstructured data, match the details about a particular entity, and build context around it. Only then can they analyze the data to gain the most value from it in their AI deployments.
4. A single view of the customer
Stitching together data across internal and external systems to create a single view of the customer is a challenge for 17 percent of financial services companies, according to the AI Forum survey. The problem is merging data from multiple sources, which stems from working with legacy IT systems or segregated data because of organizational silos.
To use this data successfully for AI use cases, financial services companies need dynamic entity resolution to connect all the data sources around people, businesses, addresses, and products, regardless of the data quality. Dynamic entity resolution uses network analysis to determine which data to use and which data to review manually. By using the combined information, financial services companies can create a trusted profile of a customer for deeper insights from their AI deployments.
5. Strong data governance, including acceptable data quality
In the AI Forum survey, 14 percent of respondents indicated the need for acceptable data quality to meet regulatory and organizational governance requirements. Data quality issues start at data entry points and become further complicated by validation shortcuts. Despite regulatory drivers for companies to also meet audit points, manual efforts by multiple teams to reconcile records can be costly.
To take control of their data quality for AI deployments, financial companies can use automated tools to build a single view of their customer records and create a candidate record based on matching rules. Then, with an analytics framework and data exploration, they can quickly find and resolve conflicts in the data.
6. Enrichment through third-party data
Financial companies struggle to create a single customer view with traditional master data management (MDM) solutions. This situation poses a real challenge for 14 percent of the finance professionals who responded to the AI Forum survey. These MDM solutions rely on probabilistic matching engines that use an algorithm to evaluate records or record scores to decide whether they match. They’re unable to pull in data enrichment from third-party data sources.
By augmenting MDM solutions with entity resolution, financial services companies get a better quality, merged customer record with more information than they can get with traditional MDM alone. The entity resolution method quickly creates a rich single view of the customer from diverse sources, enhancing its quality for greater insights.
Make the move to data readiness
Data readiness enables AI solutions to analyze, explore, and learn from data to influence and guide the decision-making processes of financial services companies. As they prepare to move to AI, they must first establish quality data by overcoming the challenges highlighted in this post. Regardless of the challenge, entity resolution, network generation, and analysis enable rich, meaningful, and contextual data for faster, trusted decisions.
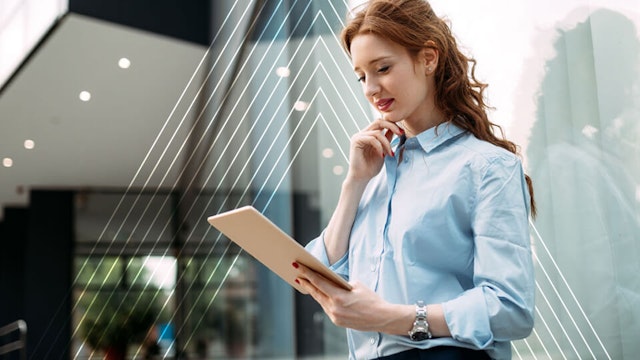