4 Signs Your Data Lake Needs a Life Raft
See how Decision Intelligence can help your teams make faster, more accurate decisions by putting your data in context.
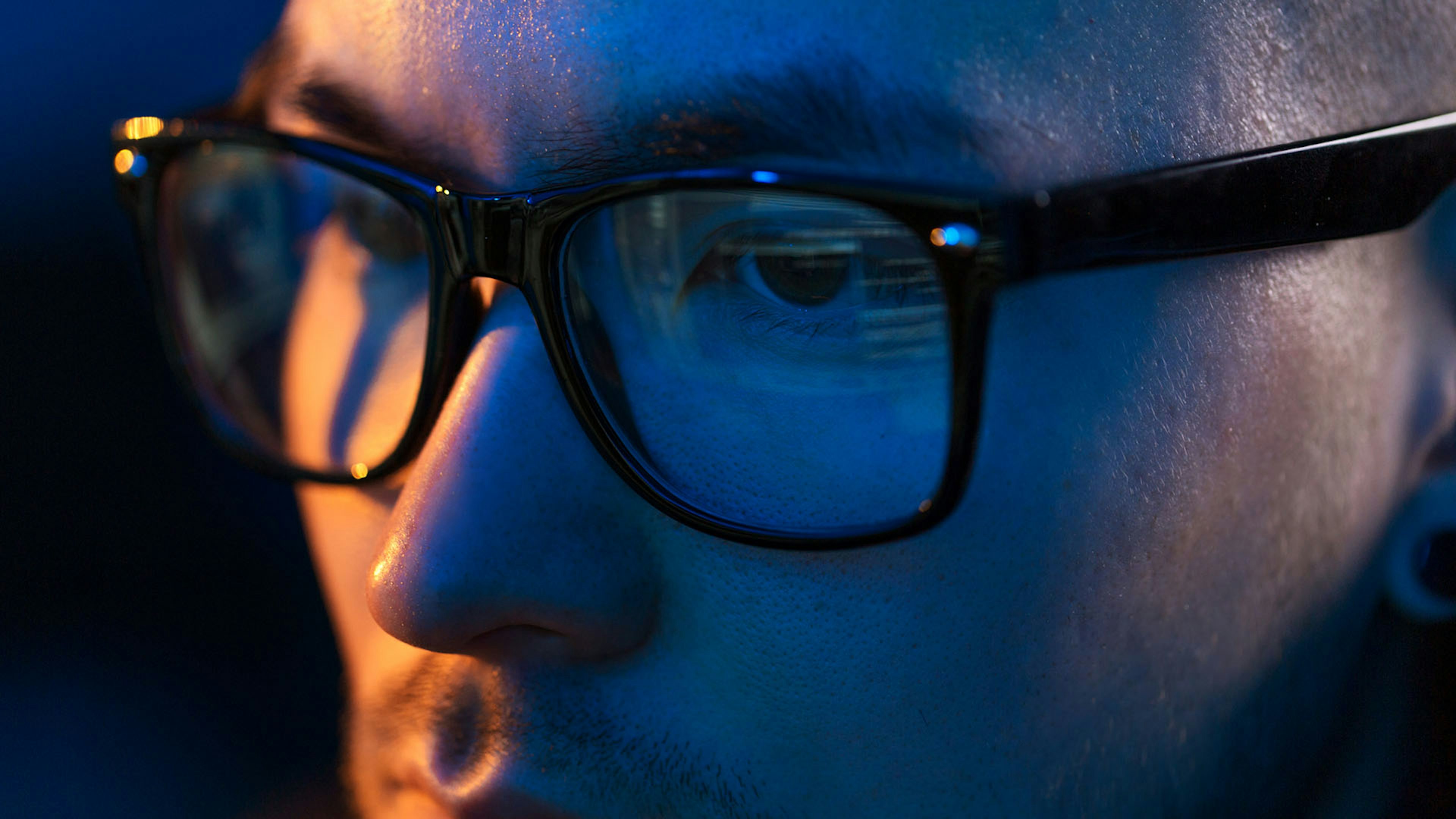
The data in your data lakes plays a key role in your company’s success. It helps provide greater value to your customers, generates insights to fuel business decisions, and creates differentiation to stay competitive. At least, that’s the promise of data lakes. Without the right resources to efficiently aggregate and process your raw data, you’re missing out on transparent and accurate data to develop value, insights, and opportunities.
Learn the four signs that the data in your data lakes needs a life raft. Then, see how Quantexa can rescue your data to keep your business sailing ahead.
#1. Your data lake has become a data dumping ground
Companies rely on data lakes to connect to and process high quantities of data in a large cluster. The data can come from multiple source systems, organizations, and even third parties. To enable their data scientists to gain insights, companies keep separate copies of the raw data from the source database, or streamed-in data for each of the major systems.
By combining all that raw data into the data lake, it becomes a dumping ground. If you have multiple sources of raw data, you must sort through them and make sense of a tremendous variety of data. Unfortunately, most data consumers are left picking through the scraps, without getting any value from across those sources.
#2. Your data scientists and engineers are data wranglers
To gain insights from the raw data, organizations must combine their data sources in some way within the data lake. They need to create a single view of their data records, which is where many organizations struggle.
If your data scientists or engineers don’t have a single view of data, they’ll try to convert your data from the original format into one they want for a task. They become “data wranglers” — cleaning and modifying data to combine it. To wrangle the data, they might use hand-coding or extract-transform-load (ETL) tools. But they don’t always get the format they need. And data that’s combined for one purpose often isn’t reusable for other tasks.
Data wrangling is an inefficient use of your data scientists’ knowledge and skills. Instead of spending extraordinary amounts of time trying to configure your data, their expertise is much better spent on analyzing a previously prepared single view of data and creating insights to drive your organization.
#3. You’re unable to aggregate your data
IT applications often store different customer, address, and transaction records. A company might keep a copy of each of those records in its data lake. Because the data isn’t aggregated, their teams must stitch it together for their reporting, dashboards, and other analytics purposes.
Your ability to stitch your data depends on the format of the raw data in your system. If you’re working on modeling or scoring for risk purposes, for example, you’re likely to spend more time sorting through data quality issues alone. Because of these data quality issues, and the time it takes to resolve them, it’s difficult to aggregate your data properly. Your time would be better spent if you could analyze data that was already aggregated to gain the insights and added value you need.
#4. Your data lake can't deliver operational data
Data lakes are based on distributed storage and processing technologies, such as Hadoop and Spark. However, data lakes aren’t operational. If business applications need data, you must move it into operational data technology, because data lakes aren’t geared toward serving data to applications.
Data that’s moved for application usage often results in multiple batch-based pipelines, where data is pushed out ad hoc. This approach can become complex and create dependency on a non-operational technology.
Enter entity resolution — the life raft for your data
The first key to these data lake challenges is finding the connections between your records, and joining the ones that are the same — a process referred to as entity resolution. The second key is to create an information profile, such as for a customer, from multiple sources. This process is referred to as network generation.
Quantexa provides both solutions in a batch environment, using Apache Spark, and in an operational environment, using Kafka and Elasticsearch. This dual architecture sets Quantexa apart from other approaches. Data is joined up in the data lake for large-scale batch or operationally using data streaming. Together, Entity Resolution and network generation work as a single data utility that serves context-rich data to any consumer.
Maximize the value of your data
Your data is your greatest asset, and one you can’t afford to lose out on. Get the most out of the data in your data lakes with the Quantexa data utility. Its Entity Resolution capabilities provide accuracy in matching and combining records. This is also scalable, as demonstrated by its ability to process billions of input records. Because it doesn’t rely on black-box techniques, the data is joined with transparent, human-readable rules to meet regulatory standards.
Plus, the network generation capabilities provide a data fabric, allowing cross-data source graph queries, either at huge scale in batch, or on demand. They enable you to create graphs from distributed data sets, including enrichment from third-party sources. The ability to combine data across systems and networks and create single, accurate profiles is unique to Quantexa.
Now that you know the four signs that the data in your data lake needs rescuing, count on Quantexa to help rescue it.
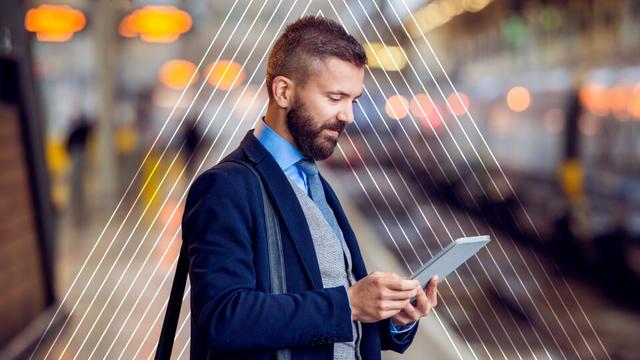