6 Ways to Boost Your ROI on AI
Generating ROI from AI starts with data quality. These proven methods help you use it most effectively.
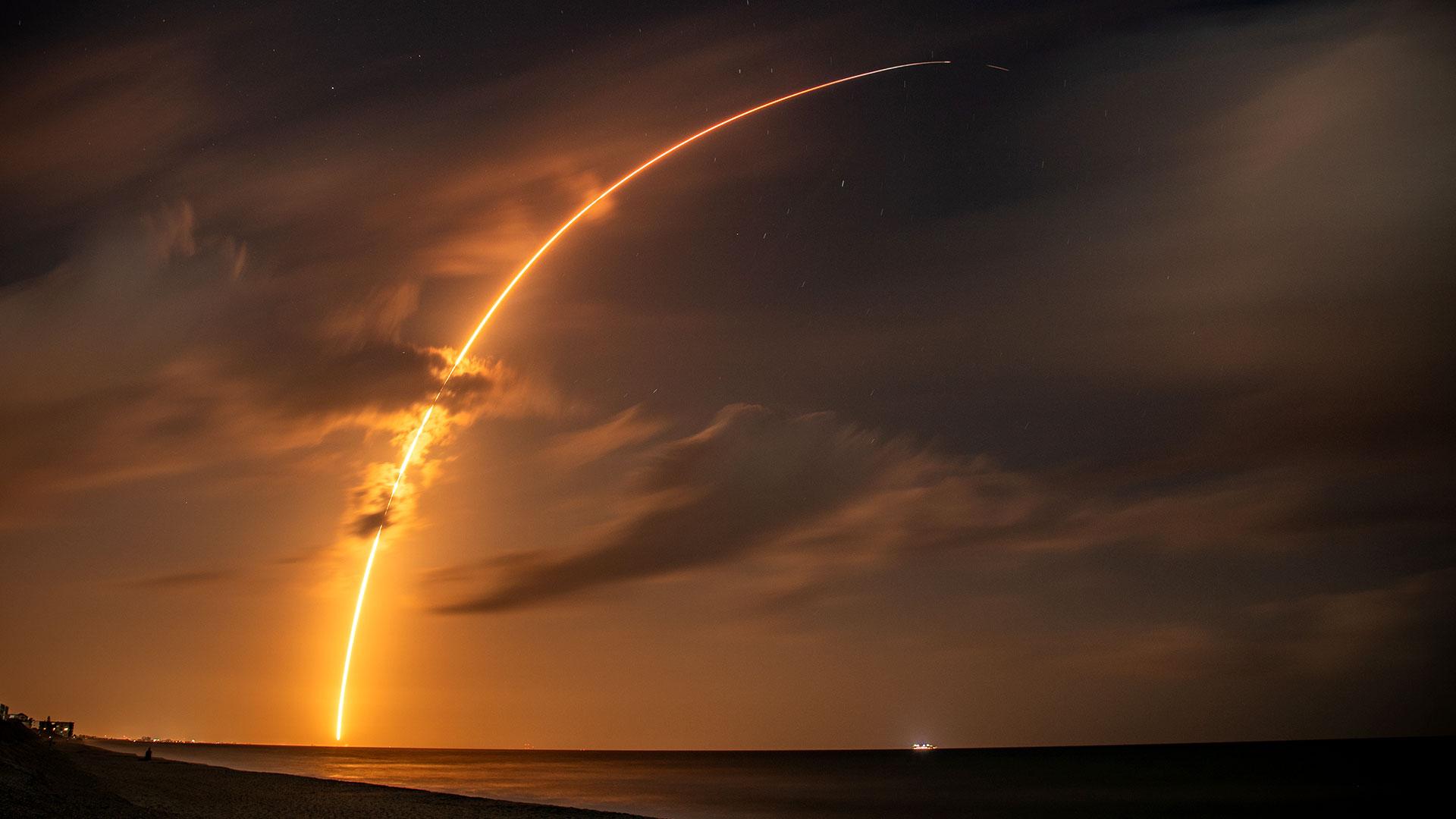
If your Artificial Intelligence (AI) goals seem out of reach, think again. All you need is the right strategy. According to a February 2022 survey by AI Forum, almost 70% of financial services professionals surveyed reported good, strong, or outstanding Return on Investment (ROI) on their AI investment. How did they do it?
Increasing ROI on AI for financial services starts with the right data, strategy, and project-specific key performance indicators (KPIs). Learning how to bring these critical areas together is key to gaining successful ROI from your AI deployment. Anything less will set organizations up for failure.
Common deployment approaches
With more at stake to meet data privacy, security, and regulatory requirements, financial services companies must determine the best AI deployment approach to meet their needs. In the AI Forum survey, financial professionals highlighted the following common approaches for their AI projects:
Buy an end-to-end solution—15%
Build their own solution—29%
Take a hybrid approach—53%
Why AI deployments fail
The reason financial services companies don’t achieve successful ROI on their AI deployments isn’t because of the approach they use. It’s due to the problems surrounding their data.
Data quality: From online forms to customer data inputs, data entry points are the start of data quality challenges. Most of these problems are caused by human error. Despite validation efforts, human error persists as employees often enter dummy text to “fool” the system. They don’t always realize the full extent of the quality issues until they combine their data with data from various customer systems.
Data management: Companies often create data lakes to manage and process their data, only to find them becoming a dumping ground of raw data. With no single view of the data, data scientists and engineers don’t get the format they need for their purpose without doing manual coding or using extract-transform-load (ETL) tools. Despite all their efforts in formatting the data, they can’t reuse the format for other tasks, requiring them to repeat the same labor-intensive processes each time.
Organizational silos: Segregating data into organizational silos hinders visibility and clarity across departments and divisions within the same financial services company. It creates a narrow view of customers, dismissing their broader relationships. Efforts to break down these silos with customer data platforms (CDPs), customer relationship management (CRM) systems, and data lakes lack the sophisticated technology to define and visualize relationships in the data.
Industry regulation and compliance: Industry regulations for financial services companies—such as Know Your Customer (KYC) and financial crime compliance—ensure an accurate view of finances and business operations. And more are on the way for AI. Starting in 2022, companies in the US and UK—with the EU just behind them—must demonstrate transparency and oversight into the use of algorithms and data sets for AI projects. Meeting regulations is difficult for financial companies without effective data quality and management processes in place. Without such processes, they risk big-time fines that can cut funds and resources for future AI projects.
6-step AI deployment strategy for successful ROI
Data aside, achieving successful outcomes from your AI projects demands the right strategy. It requires taking in a big-picture view to understand where, how, and why to implement AI across the enterprise.
While historically, lackluster ROI in AI has troubled the financial services sector, 68% of financial services professionals have indicated good or even strong ROI from their AI initiatives due to the implementation of a successful AI deployment strategy.
To follow suit, start by following this six-point strategy for a successful ROI of your AI project.
1. Find the right problems
Investing in AI for financial services isn’t a one-and-done project. Rather, it entails identifying problems across your company that can benefit from AI. For example, it can mean opportunities for:
Reducing time-intensive and resource-intensive costs
Offering a better customer experience
Providing advanced fraud detection
Improving the ability to meet regulatory compliance
Refining loan and credit decisions
Automating investment research and guidance
These challenges occur in various areas across the organization, and not necessarily at the same time. By understanding the problems to address up front, you’ll know what data you need and which sources to pull from.
2. Define your business use case
Once you know the problem(s) you want to solve using AI, decide on a business use case. Financial services companies often use AI for:
Business development
Compliance, surveillance, and anti-money laundering
Credit risk decisioning
CRM, customer service, and customer insight
Financial crime and fraud detection
Governance and risk
KYC and customer onboarding
Operational optimization
Defining your use case requires meeting with key stakeholders from your business operations, technology, compliance, and data science teams to identify:
Repetitive, time-consuming tasks that rely on data and rules
Critical business information hidden in your data that you need to access
Areas where human decision-making can create context around data
Unstructured data that’s used, accessed, and served
Ways your internal and external customers use enterprise applications
By getting buy-in from all departments, enterprises can build a solid foundation for their use case and show upper level management that the company is on track to meet their AI goals.
3. Clean up your data
While this step might be third in this list, it just might be the most critical foundational step for achieving AI success. AI runs on big data to generate business and customer insights. But to be successful, you need to be data-ready so you can create a single, connected customer view to create context around your data. Data lakes, data silos, CDPs, and CRMs don’t allow for this level of quality to enable AI decision-making.
Data readiness requires:
Entity Resolution to connect your data from across your enterprise
Network generation to build a dynamic, big-picture view of your internal and external data
Advanced analytics to build analytic models based on real-world experience
Network visualization to create context around your data for better decision-making
By establishing this level of data quality across your enterprise, leaders can make more meaningful and trusted decisions.
4. Address security, privacy, and regulatory issues
Taking on AI means your project must meet regulatory requirements, particularly for data security and privacy. Brushing over these areas puts your entire enterprise at risk for a data breach, lost or stolen data, and, potentially, millions of dollars in fines by industry and government regulators.
To address these challenges, enterprises must:
Create a big-picture view of your data through Entity Resolution and network generation.
Establish the necessary data protection measures across the enterprise:
Minimizing human error
Fine-tuning data handling procedures
Securing your technical infrastructure
Applying the right mix of security tools, skills, and expertise
Stay current on industry and federal regulations to uphold data security and privacy.
By safeguarding your data this way, you can guard against fraudulent activities and maintain your data confidentiality, integrity, and privacy.
5. Implement your program one step at a time
AI projects often fail because companies try to do too much at once by rushing to deployment. Successful AI deployment requires careful planning and the right mix of resources:
Build a cross-functional group of representatives from your data science, business analyst, business professional, development, data protection, security, and IT teams
Identify the skills and resources you need and have, then address and fill any gaps
Make sure everyone knows their roles and expectations for meeting the project’s goals
Develop a timeline that keeps the implementation moving smoothly
Allow for open communication and transparency along the way
With this basic guide, your AI deployment will get off to a good start and achieve successful deployment.
6. Measure the benefits from your AI deployment
The true test of successful ROI—hard ROI—on your AI deployment is in your project’s KPIs. For example, you might evaluate:
Time savings earned by automating time-consuming, manual, or cognitive tasks
Productivity gains by investing in AI for more effective and efficient processes
Cost savings realized by implementing AI
Break-even point of when the cost savings equals your investment into the project and the time to reach that point
Revenue growth from new products and services enabled by AI
Also, consider the soft ROI, such as:
Improved productivity
Better customer experience
Skills retention
Greater agility in taking on new opportunities and challenges
By measuring both hard and soft ROI, you can more accurately gauge the success of your AI deployment.
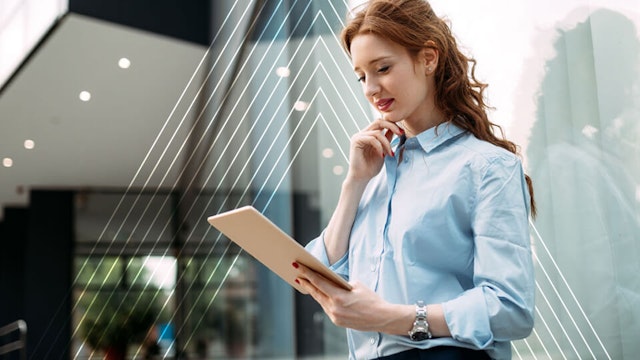