How Insurers Are Boosting Their Fraud Detection To Stop Criminals
Insurers can stay ahead of the curve when it comes to fighting tech-savvy fraudsters using advanced analytics.
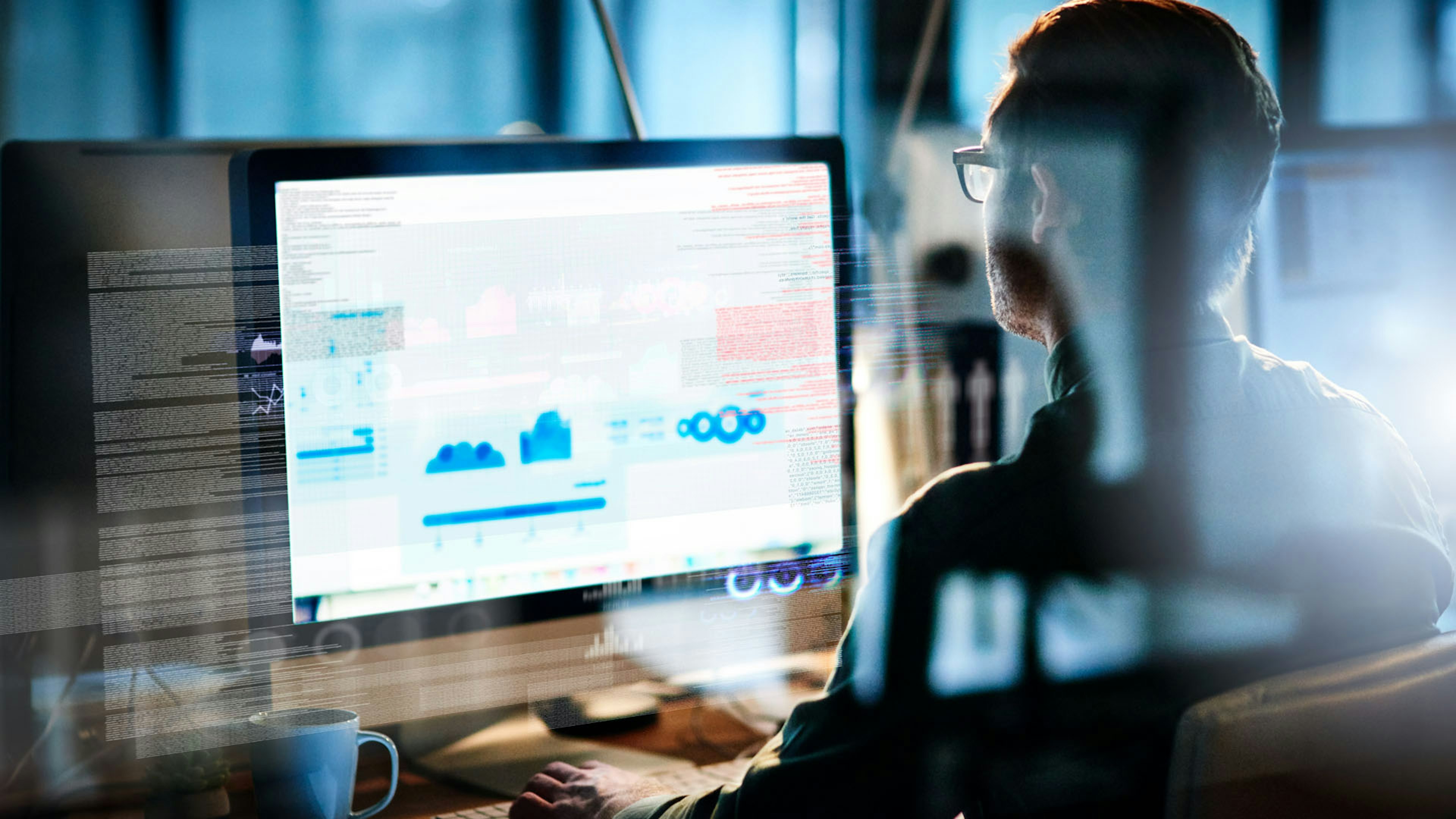
Insurance fraud is a well-known and long-established problem. It has many guises and ranges from small one-off opportunistic cases to multi-million dollar syndicates of customers and suppliers working together to routinely defraud the insurer.
Some of the lessons learned in Europe and the US are yet to head East, and while the fraudsters are dining out on their well-executed crimes, the average punter is picking up the bill by paying high premiums to offset the fraud losses. The current estimates are that the cost of insurance fraud to the industry is $80bn per annum. Some believe the true figure is even higher.
However, it's not all doom and gloom. The Australian insurance industry is faced with a fantastic opportunity to become a global center of excellence in the fight against insurance fraud. By learning from the successes and failures seen in other parts of the world and leveraging recent technological innovations, Australian insurers are now able to fight back, stem the losses, and stop allowing criminals to impact policy pricing and customer experience.
The beginning of an insurance fraud detection strategy
Within the Australian insurance industry, there is a broad spectrum of fraud detection and investigation mechanisms in operation. There are still plenty of insurers out there who rely on tip-offs and the gut instinct of claims handlers to detect fraud, whilst others are using basic automated business rules and scorecards, and a small handful are adopting more advanced techniques.
While there is a collective moral obligation for the industry to stamp out crime, those who do it most effectively have an opportunity to out-compete those who are behind the curve, through sharper policy pricing and a better-claiming experience for the customer.
Only by bringing multiple techniques together and accounting for the interconnected nature of human behavior, can insurers enable an effective detection strategy that stands up to the sophistication of modern criminals, whilst still addressing the high volumes of low-value opportunistic events that chip away at the bottom line. Moving away from lengthy batch-based processing and embracing real-time also helps insurers to keep a critical eye on customer experience.
Events to entities to networks: A fraud detection maturity curve
Insurance fraud detection is a data analytics problem, all about trying to use data to identify and act on patterns of human behavior. To understand fraud, or any other behavior, it is vital that humans (and their businesses) are analyzed as they exist in the real world: as holistic, interconnected entities living and operating in social groups with other people, businesses and material things. Crucially, these relationships are not static. They are constantly changing, and detection approaches must account for this dynamic paradigm by processing high volumes of data in real-time and enabling detection models to evolve with clever criminals.
Detection strategies must be able to generate and constantly update a view of entities as they exist in the real world, and to apply a scoring model (comprised of business rules and analytical techniques) to the full picture. This includes events (such as a claim), entities (such as a customer or supplier) and networks (such as groups of customers who are connected). If claim scoring accounts for all these dimensions, detection rates increase significantly. Capturing investigative outcomes and leveraging AI to make enhancements to detection models is also vital for detection strategies to remain sharp.
It has been proven that adding entity and network variables to a detection strategy significantly increases detection rates, reduces false positives and brings the complex, hidden & multi-faceted cases to the surface. To achieve the best outcomes, human beings must be analyzed as they exist in the real world.
So why isn't everyone doing it?
The limitations of fraud detection... until now
Whilst simply adding entity and network variables to a detection strategy may sound like a simple task, the complexity comes from how difficult it is to generate an accurate single view of a customer in the first place. Even worse is attempting to automatically identify the meaningful links that the customer has with other people and businesses in the data. To enable a sound detection strategy, though, this view must first be generated, even in the face of incomplete, poor-quality data coming from a multitude of different systems.
It is also critical to bear in mind that sophisticated fraudsters purposefully manipulate their identities, which will change from one policy or claim to the next. Entity Resolution, which is the process of generating a single view of an entity from multiple data sources, must cater to this manipulation.
Only once an accurate single view of entities has been created, can the relationships between them be identified. Again, this is no simple task. Consider the"six degrees of separation" rule which posits that any one person in the world is connected to any other by six or fewer steps. In any insurer, especially one with a large market share of a population, everyone is likely to be connected to everyone else, somehow.
The art is in using a combination of rules and statistical techniques to identify the meaningful links and suppress the insignificant ones. This allows accurate networks of people, businesses and other entities, which are genuinely connected in the real world, to emerge from the data, laying the foundation for the sophisticated detection models required to catch sophisticated fraudsters.
Advances in open source technology now allow this to be done live, in real-time, and on-demand, minimizing disruption to the customer's claiming experience and delivering to-the-minute insights.
How to develop an effective fraud detection engine
Using the latest open-source platforms to develop a highly accurate and flexible insurance fraud detection and investigation capability will enable insurers to tackle the challenges around insurance fraud head-on.
The following capabilities are some of the ways to help make this achievable:
Dynamic Entity Resolution – The ability to generate a true single view of customers, suppliers and other entities, on the fly to to allow more information to come in and out of the network view
Graph Analytics – Automatically identify the meaningful links between entities and suppressing the insignificant ones
Scoring networks – Fraud detection models which can be tweaked for each insurer, market and data set, and developed over time. Crucially, these will take event, entity and network level variables into consideration to maximise detection rates
AI – Machine learning can be used to tune detection models over time, keeping them relevant
To find out more about how to identify potentially fraudulent activity faster, visit our solutions page.
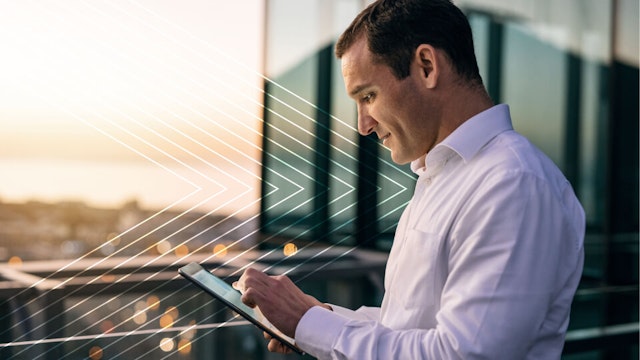